1. Intro:
Artificial intelligence (AI) and machine learning (ML) have become household buzzwords across virtually every domain of science and technology. Beyond the academic sphere, the advent of open-source AI tools such as ChatGPT and Bard has quickly spread the notion that there is little AI cannot do. Indeed, AI applications have now become commonplace in many aspects of day-to-day life, from web search engines to image and speech recognition software.
In the field of drug discovery, AI has also received a lot of attention from biotech and big pharma companies, with several AI-designed drug candidates making it through to the clinic in recent years (Table 1). Morgan Stanley predicted in 2022 that AI-enabled improvements in early-stage drug development could represent a potential $50 billion boost to the market over the next decade1. Third-party investment in AI-enabled drug discovery reached $5.2 billion at the end of 2021, already more than double the $2.4 billion figure attained in 20202. CPHI’s Annual Report 2023 for the first time reveals that pharmaceutical ‘AI companies’ have overtaken ‘late stage’ as the industry’s most appealing investment option for venture capital (VCs)3. Clearly, the AI/ML drug discovery field is entering a boom period in terms of funding. But is it all plain sailing from now on, or do there remain substantial questions over the successful implementation of AI in drug discovery?
1.1. The need for AI in drug discovery:
Bringing a drug to the market is becoming an ever more costly endeavor for pharma companies. In 2022, it was estimated that the average cost of R&D for the top 12 biopharma companies was $2.284 billion per drug – double the $1.188 billion calculated in 20104,5. Around one-third of that figure is spent on the drug discovery phase, a phase that can last 5-6 years before a candidate is taken forward to clinical trials5. Over the same period, forecast peak sales per late-stage asset in the pipeline have more than halved from $816 billion to $389 million4,5. The subsequent expected return on investment has therefore decreased from 10.1% in 2010 to 1.2% in 20224,5.
With a typical development timeline of over 10 years and a clinical failure rate of 90%, which has not changed much over the years, there is clearly a need to streamline the drug discovery process and improve the probability of success in reaching and achieving drug approvals. Consequently, computational approaches aimed at avoiding time-consuming experiments at the early stage of drug discovery could considerably enhance the process, provided the quality of the outcome is maintained, or ideally, improved.
1.2. Computational approaches in drug discovery are not new:
The use of computational methods in drug discovery is not a recent development. In 1981, Fortune magazine hailed computer-aided drug design (CADD) as the next industrial revolution6. CADD refers mostly to structure- and ligand-based approaches in pre-clinical drug discovery, including molecular docking, virtual screening, and quantitative structure-activity relationship (QSAR) among others. While CADD has experienced its fair share of hype and disillusionment, it has now become a mainstay to help identify suitable lead molecules for most preclinical programs. This is predominantly thanks to an ever-increasing repertoire of 3D protein structures, a rapid expansion of drug-like chemical space, and the advances in cloud and graphics processing unit (GPU) computing resources7.
Machine learning (ML) is not new to the field either. In 1994, QSAR modeling was established as a method to predict phenotypic effects such as toxicity by deriving equations from focused subsets of compounds8. This led to more in-depth research aimed at capturing properties such as pharmacophores and three-dimensional structure. The structural revolution in combination with an increasing amount of data from high-throughput screening further paved the way for more complex ML models in the 21st century.
The terms AI, ML and deep learning (DL) are often used interchangeably but it's worth defining them individually. AI is an umbrella term that refers to the use of technologies to build machines and computers that have the ability to mimic cognitive functions associated with human intelligence, such as analysing data and making recommendations in the context of drug discovery. ML, on the other hand, refers to a subset of AI that automatically enables a machine or system to learn and improve from experience in a process known as training, where algorithms are exposed to training data as part of the development process9. DL can be thought of as a further subset of ML that uses more complex artificial neural networks to process data through various layers of algorithms and find accurate solutions without human intervention (Figure 1).
Figure 1: Distinguishing the terms artificial intelligence (AI), machine learning (ML) and deep learning (DL).
It wasn’t until 2013 that complex DL methods finally demonstrated real benefit over other ML approaches. That year, a SAR challenge set by Merck was won by a team that used a deep neural net to make better prospective predictions than random forests (an ML algorithm) on a set of large diverse QSAR data sets10. The rapid growth and public availability of “big data” stored in chemical databases such as PubChem and ChEMBL further supported model training and validation, allowing for more accurate AI medicinal chemistry tools11.
Suffice to say that over the past decade, developments in AI-facilitated drug discovery have sky rocketed, and an increasing amount of attention has turned towards computational approaches in industry. The rapid progress of AI is in part due to the immense computational power now at our disposal. Research by OpenAI showed that between 2012 and 2018, the amount of ‘computing power’ used in the largest AI training runs increased exponentially with a 3.4-month doubling, a growth that corresponded to a 300,000-fold improvement12. Now, nearly every stage of the drug discovery pipeline has now been targeted with a relevant AI tool, and the fruits of this labour are now starting to be seen, with over 100 submissions across drug and biological product applications mentioning AI/ML in 202113. Here we consider the various applications of AI/ML in drug discovery and assess potential limitations as well as future directions in the field.
2. Promising contributions of AI to drug discovery:
2.1. Target identification:
Target identification is a crucial and often time-consuming step in drug discovery that strongly influences the probability of success at every step of drug development. It involves the identification of the right biological molecules or cellular pathways that can be modulated by drugs to achieve therapeutic benefits. The combination of multi-omics (e.g. genomics, epigenomics, proteomics) with experimental and computational approaches forms the basis of target identification. However, the overwhelming amounts of biomedical data amassed from diverse sources such as fundamental research on disease mechanisms and patient-derived data have made data analysis an immensely complex task. This is particularly the case in complex diseases where the underlying disease mechanisms are poorly understood14.
One recent example is amyotrophic lateral sclerosis (ALS), a severe neurodegenerative disease with poorly defined pathogenesis. In 2022, Pun et al. applied an AI-based target discovery platform PandaOmics to analyse the expression profiles of central nervous system (CNS) samples and direct induced pluripotent stem cell (iPSC)-derived motor neurons, resulting in the discovery of 28 AI-proposed targets for ALS treatment. These targets were subsequently validated in an ALS-mimicking Drosophila model, in which the suppression of eight unreported targets strongly attenuated eye neurodegeneration15.
Besides the complex multi-omic data crunching approach, AI image recognition algorithms have also been implemented at the target identification level. Yang et al. incorporated an image-based deep learning method to identify cardioprotective small molecules in a phenotypic screen using human iPSC-cardiomyocytes (iPSC-CMs)16. In this case, the neural networks were trained using approximately 1,300 immunocytochemistry images from both BAG3-depleted and control iPSC-CMs, resulting in a model that could successfully separate both classes of cells with 95% accuracy. Fifteen HDAC inhibitors were identified from a screen of 5,500 bioactive compounds that significantly protected the iPSC-CMs from sarcomere damage. HDAC6 in particular was validated as a suitable target for dilated cardiomyopathy (DCM) after reducing sarcomere damage in mouse models, and awaits further preclinical work17.
2.2. Protein structure prediction:
Once a protein target has been identified, considerable efforts are typically made to obtain a three-dimensional structure of the protein, ideally in a biologically relevant orientation, if it is not already available. These efforts are often run in parallel to primary screening experiments so that any promising hits can be validated structurally. If the medicinal chemist knows how and where a hit binds to the protein target, then the process of hit-to-lead optimization can be facilitated through the rational, structurally-enabled design of novel molecules that bind optimally to the target site18. The problem is that in many cases, obtaining 3D structures of protein-ligand complexes is not straightforward and often involves techniques such as X-ray crystallography and cryo-electron microscopy (cryo-EM). These techniques have their limitations (as discussed elsewhere19,20), meaning that several drug discovery projects involving proteins that do not readily crystallize, or are too small for cryo-EM, are left ‘blinded’ by a lack of structural data to support chemists during the rational design process.
Over the past 40 years, the number of structures solved experimentally and deposited in the Protein Data Bank (PDB) has grown exponentially, with over 200,000 structures now present, covering the whole spectrum of protein classes[21]. Fueled by this growth in the PDB, computational efforts aimed at solving the ‘protein folding problem’ have accelerated in recent years. Despite much progress being made using both computational physics and bioinformatics approaches, it wasn’t until 2020 that the first computational method was reported that could predict protein structures to near experimental accuracy. This much-acclaimed breakthrough came at the 14th Critical Assessment of protein Structure Prediction (CASP14) when Google Deepmind presented their neural network-based model, AlphaFold2[22]. By training the algorithm on the hundreds of thousands of protein structures and sequences in the PDB, AlphaFold2 could accurately predict structures from the amino acid sequence with a median backbone accuracy of 0.96 Å root mean square deviation (RMSD), compared to the next best performing method which had a backbone accuracy of 2.4 Å RMSD22.
One week after the breakthrough publication in July 2021, DeepMind announced that it had used AlphaFold to predict the structure of nearly every human protein, in addition to proteins present in other well-studied organisms, comprising 365,000 structures in total23. One year later, the structures of around 200 million proteins had been predicted by AlphaFold, with the European Molecular Biology Laboratory’s European Bioinformatics Institute (EMBL-EBI) deeming that 35% of those predicted structures were as good as experimentally determined structures24. Such unprecedented speed and accuracy earned AlphaFold2 widespread acclaim and led many to believe that it would transform drug discovery, allowing researchers to bypass the time-consuming and arduous task of experimentally determining the structure of protein-ligand complexes.
However, the step-up from apo-protein structure prediction to protein-ligand complex structure prediction is not as simple as it seems. Karelina et al. reported in May 2023 that the accuracy of ligand binding poses predicted by computational docking to AlphaFold2 protein structures was much lower than when docking to experimentally determined apo-protein structures25. The reasons for this are still unclear but may be due to small differences in the orientation of side chains between the experimental and predicted structures. Nevertheless, Recursion recently announced that it had used its AI tool MatchMaker to calculate the protein targets of 36 billion drug-like compounds in the Enamine Real Space library, screened against 15,000 human protein structures predicted by AlphaFold26. MatchMaker is a neural network trained on drug-binding pockets from over 8000 PDB and SwissModel co-complex structures to discriminate bioactive drug-target pairs from randomized pairs[27]. While this method was shown by Recursion to facilitate the discovery of a novel DCAF1 ligand, it did not predict the binding poses of the screened ligands, a different challenge altogether that has also been tackled by various computational approaches over the years.
2.3. Virtual screening:
The recent expansion of chemical libraries containing billions of molecules that can be synthesised on demand, such as Enamine Real Space, Mcule ultimate, and WuXi GalaXi, has strongly encouraged the growth of ultra-high throughput virtual screening methods28. In a conventional high-throughput screen, libraries of 50,000 – 500,000 compounds are screened against a given target, often leading to only a handful of ‘hits’ after secondary validation. However, these hits often exhibit relatively poor and non-selective target binding affinity and can have suboptimal ADMET and PK properties. It then takes several years of hit-to-lead optimization to reach a lead molecule with suitable properties for clinical progression.
Meanwhile, conventional virtual screening efforts have been limited to screening fewer than 10 million compounds before in vitro validation, offering only a marginal scale advantage over the top HTS screens. Simply scaling up the current in silico screens is not a viable solution either – it has been calculated that it would take more than 3,000 years to screen 1010 compounds on a single CPU core [7]. Similarly, simply screening larger libraries represents a real danger of amplifying false positives – even with a 0.000001% false positive hit rate, the total number of false positive hits from a screen of 10 billion compounds would be 10,000.
One example of a deep learning solution to ultra-scale virtual screening is the dockAI platform currently being developed by Iktos. This platform harnesses a branch of ML methodology called active learning, where an increasingly accurate model can be trained to act as a stand-in for a difficult or computationally costly scoring function. As a proof of concept, the docking algorithm was trained against a subset of published results from an established docking method. After iterative scoring and retraining of the ML algorithm to correctly predict docking scores, the top 5% of compounds predicted with the ML model were then docked to evaluate the accuracy of the model. More than 80% of the experimentally confirmed hits were successfully recovered by the ML method with a 14-fold improvement in computing cost28. In March 2023, Iktos announced the closure of a €15.5m Series A financing, while also maintaining collaborations with several big pharma companies.
Similarly, recent work at MIT’s Jameel Clinic has led to the development of DiffDock, a diffusion generative AI model (DGM) that can carry out molecular docking with fast inference times and high selective accuracy. Instead of taking a classical “sampling and scoring” approach, where ligand poses are searched that best fit the protein pocket, DiffDock takes a “blind docking” DGM approach. This involves first training the model on a variety of protein-ligand poses, where a gradual noising process helps grow a neural network that can then recover, or denoise, the protein-ligand poses29. Faced with protein and ligand structures outside of the training set, DiffDock is then able to denoise randomly sampled ligand poses via a reverse diffusion over translational, rotational, and torsional degrees of freedom. These sampled poses are then ranked by the confidence model to produce a final prediction and confidence score30. On the PDBBind blind docking benchmark test, DiffDock achieved a 38% top-1 prediction with RMSD < 2 Å, considerably surpassing the previous best search-based (23%) and deep learning methods (20%).
AI-accelerated protein-ligand docking is still a relatively recent addition to the field, with various publications reporting the development of alternative AI docking methodologies over the past two years7,31,32 . It remains to be seen to what extent these DL-based methods will become commonplace in the field of drug discovery. It is likely that the ability to accurately dock ligands onto computationally generated protein structures will be one of the next objectives in the field. Besides DiffDock, existing state-of-the-art molecular docking tools fall short, and perform only a little better than chance[33,34].
2.4. Physicochemical and ADMET properties:
The physicochemical properties of a drug candidate can have a huge influence on the likelihood of clinical progression. Understanding the pharmacokinetic and pharmacodynamic (PK/PD) profile of a drug is crucial in the clinic, but the properties governing the PK/PD profile can often be difficult to predict and optimize. QSAR modeling in particular aims to find a mathematical relationship between molecular properties of a compound and its biological activity. QSAR algorithms are typically trained on data obtained for a given target and a chemical scaffold with the goal of guiding the optimization of affinity and potency. Unsurprisingly then, the quality of the QSAR model is highly contingent on the quality and availability of the data obtained for a given target class.
ML approaches have been used for over 20 years to facilitate QSAR modeling. Methods based on linear regression, Bayesian neural networks and random forests (RF) have been reported, with RF being the most commonly used algorithm34. Neural networks were used for QSAR in the 1990s, however, due to the high prediction accuracy, ease of use and robustness of RF, neural networks quickly lost their place to RF as the gold-standard method35. The watershed moment for deep neural networks came in 2012 when a Merck-sponsored Kaggle competition focusing on QSAR problems was won by a team utilizing a deep neural net (DNN). The crucial difference between the neural networks used in the 1990s and the DNNs used ubiquitously across current AI applications is that the DNNs have more than one intermediate or ‘hidden’ layer and more neurons in each layer, resulting in both deeper and wider networks (Figure 2)7. In the past, neural networks were limited to a small number of input descriptors and were prone to overfitting, modern DNNs with multiple hidden layers and thousands of neurons in each layer can now face datasets with hundreds of thousands of compounds and thousands of descriptors.
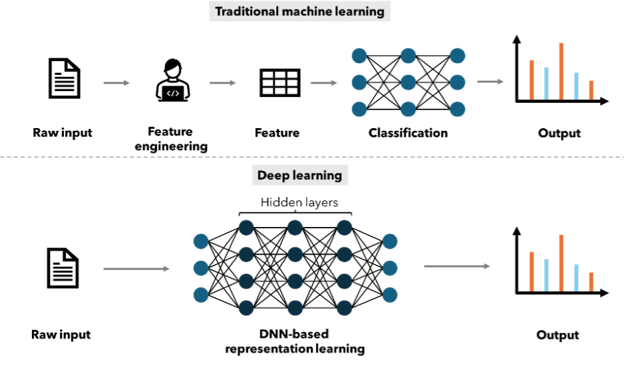
Figure 2: Schematic overview of the differences between traditional machine learning methods and deep learning methods.
However, SARs are notoriously complex, giving rise to the SAR paradox or activity cliffs – the observation that some molecules with highly similar structures can exhibit large differences in potency. In a 2022 publication, van Tilborg et al. highlighted the noticeably poor performance of deep learning approaches to correctly anticipate cliff compounds, demonstrating the need to include structure-activity disconnects during model training and selection[36]. Similarly, DL-based QSAR algorithms failed to outperform simpler kernel regression models in a QSAR binding prediction challenge focusing on the kinase superfamily37. Given the broad availability of data for protein classes and superfamilies such as kinases and aminergic GPCRs, the quality of simpler QSAR algorithms can often be more than sufficient. It remains to be seen how well DL QSAR algorithms perform for other smaller protein families.
2.5. De novo drug design:
One step beyond in silico screening and QSAR modeling is the ability to design de novo drug candidates with desired efficacy, safety, and PK/PD profiles. Compared to virtual screening, AI-assisted de novo design can generate a wider variety of structures without the need to recourse to pre-existing compound libraries. In 2019, at least nine AI tech providers were starting to offer tailored de novo drug design services4. However, de novo drug design is understandably much more complex than just virtual screening, and as of September 2023, only one entirely AI-designed drug, by Insilico Medicine, has successfully entered Phase 2 trials38.
Insilico Medicine is pioneering the end-to-end use of AI in drug design, with its candidate small molecule INS018_055 entering Phase 2 clinical trials in China for the treatment of idiopathic pulmonary fibrosis (IPF). The approach taken involved target identification through multi-omics and deep biology analysis, followed by automated, ML de novo drug design using its Chemistry42 platform. This platform comprises 40+ generative models that function in parallel to generate novel structures upon addition of user data. The generated molecules are filtered before being subjected to multiple sets of reward and scoring modules that assess the molecular properties according to the predefined criteria. These modules form the core of the backbone of the multiagent reinforcement learning (RL)-based generation protocol39.
Besides Insilico, companies such as Exscientia and BenevolentAI have also pioneered the use of generative AI in end-to-end drug discovery. Exscientia describes itself as a ‘full stack AI drug discovery company’ and has implemented AI tools across the discovery pipeline, from target identification to precision medicine in clinical trials. In September 2023, Merck KGaA and Exscientia signed a collaborative deal worth up to $674 million to harness the AI-driven precision drug design and discovery capabilities of Exscientia. Another collaboration with Bristol Myers Squibb worth up to $1.2 billion has also resulted in the progression of a PKC-theta small molecule inhibitor, EXS4318, to Phase 1 trials in February 202340. In this case, generative AI was used to rapidly explore selectivity-focused scaffolds and design a nominated candidate in under 11 months.
2.6. Drug repurposing:
AI/ML has not only gained traction in medicinal chemistry and drug design but also in drug repurposing, a concept that involves expansion of an approved or investigational (including clinically terminated) drug into a new therapeutic area. The ever-growing wealth of data surrounding drug candidates across a range of indications lends itself to computational approaches aimed at finding the ideal drug for a given disease. For example, the Chemiverse platform developed by Pharos iBio combines big data and AI technology to repurpose existing therapies, such as the small molecule Phi-101 that was originally developed as an acute myelogenous leukaemia (AML) therapy and is now in Phase 1 trials for the treatment of ovarian cancer.
The COVID-19 pandemic served as an ideal opportunity to demonstrate the speed and efficiency of AI approaches in identifying effective COVID-19 therapeutics through repurposing. One such example was the deep learning approach taken by Zeng et al. to rapidly identify 41 drug candidates for the potential treatment of COVID-1941. In this approach, a knowledge-graph-based deep-learning methodology was built that integrated scientific literature and drug properties from 24 million PubMed publications and the DrugBank. Of the 41 repurposable drugs identified, nine were, or had been, in clinical trials for COVID-19 at the time of publication, including corticosteroids such as dexamethasone that was shown to reduce mortality in cases of severe COVID-19 infection42. A similar knowledge graph approach was taken by BenevolentAI to identify baricitinib, at the time a rheumatoid arthritis therapy, as a candidate for treating patients with COVID-1943. Olumiant (baricitinib) was subsequently approved by the FDA for the treatment of COVID-19 in May 2022.
Several companies are now actively engaged in leveraging the abundant omics data of human disease to reposition drugs in new therapeutic areas. AstraZeneca has been developing computational approaches to repurpose drugs since 2015, and in 2020 identified complementary gene expression profiles induced by idiopathic pulmonary fibrosis (IPF) and saracatinib, a candidate drug originally developed to potentially treat cancer44. The Phase 1b/2a clinical trial of saracatinib to treat IPF is currently ongoing and is expected to reach completion at the end of 202345.
Other smaller companies such as Auransa and Healx have also developed in-house knowledge graphs to automate disease biology data mining and identify novel disease-compound relationships, often incorporating FDA-approved drugs already on the market. While the pipelines of both companies are still focusing on developing novel drug candidates, the ability to quickly find FDA-approved candidates for drug repurposing could prove crucial in emergency situations like a pandemic, where time-consuming preclinical animal safety experiments can be circumvented.
Table 1: Current clinical pipelines of ‘AI-first’ companies developing small molecules, adapted from a table published by the Wellcome Trust/BCG in June 2023 46.
Company |
Molecule Name |
Global Status |
Therapeutic Area |
BioXcel Therapeutics |
dexmedetomidine |
Launched |
Mental health |
Nimbus Therapeutics |
NDI-034858 |
Phase 3 |
Oncology |
Neumora Therapeutics |
NMRA-140 |
Phase 3 |
Mental health |
Recursion Pharmaceuticals |
REC-2282 |
Phase 2/3 |
Oncology |
A2A Pharmaceuticals |
AO-001 |
Phase 2 |
Oncology |
OrphAI Therapeutics |
apilimod dimesylate |
Phase 2 |
Covid-19 |
OrphAI Therapeutics |
AIT-101 |
Phase 2 |
Neurology |
BPGbio |
ubidecarenone |
Phase 2 |
Oncology |
HemoShear Therapeutics |
HST-5040 |
Phase 2 |
Metabolic |
Landos Biopharma |
NX-13 |
Phase 2 |
Inflammatory disease |
Nimbus Therapeutics |
firsocostat |
Phase 2 |
Oncology |
InSilico Medicine |
INS018-055 |
Phase 2 |
Respiratory |
Nobias Therapeutics |
fasoracetam |
Phase 2 |
Neurology |
Recursion Pharmaceuticals |
REC-4881 |
Phase 2 |
Oncology |
Recursion Pharmaceuticals |
REC-994 |
Phase 2 |
Neurology |
SOM Biotech |
tolcapone |
Phase 2 |
Neurology |
SOM Biotech |
bevantolol |
Phase 2 |
Neurology |
Nimbus Therapeutics |
NDI-101150 |
Phase 1/2 |
Oncology |
Accutar Biotechnology |
AC-682 |
Phase 1 |
Oncology |
Accutar Biotechnology |
AC-0176 |
Phase 1 |
Oncology |
Accutar Biotechnology |
AC-699 |
Phase 1 |
Oncology |
AI Therapeutics |
sirolimus |
Phase 1 |
Immunology |
BioAge Labs |
BGE-105 |
Phase 1 |
Musculoskeletal |
Black Diamond Therapeutics |
BDTX-1535 |
Phase 1 |
Oncology |
C4X Discovery |
INDV-2000 |
Phase 1 |
Mental health |
Exscientia |
EXS4318 |
Phase 1 |
Immunology |
Nimmune Biopharma |
LABP-104 |
Phase 1 |
Immunology |
Neumora Therapeutics |
NMRA-511 |
Phase 1 |
Neurology |
Pharos iBio |
PHI-101 |
Phase 1 |
Oncology |
Recursion Pharmaceuticals |
REC-3964 |
Phase 1 |
Infectious disease |
Relay Therapeutics |
RLY-2608 |
Phase 1 |
Oncology |
Relay Therapeutics |
RLY-4008 |
Phase 1 |
Oncology |
Schrodinger |
SGR-1505 |
Phase 1 |
Oncology |
SOM Biotech |
SOM-1311 |
Phase 1 |
Metabolic |
SOM Biotech |
prexasertib |
Phase 1 |
Covid-19 |
Verge Genomics |
VRG-50635 |
Phase 1 |
Covid-19 |
3. Limitations:
The past five years has been described as an ‘AI Spring’ given the rapid growth of generative AI platforms across all STEM domains. In the domain of drug discovery, AI tools have shown their capabilities in streamlining often time-consuming and costly stages of the discovery pipeline, such as target identification and lead optimization. Indeed, BCG estimated in 2022 that AI-driven R&D efforts at both the discovery and preclinical stages could translate to time and cost savings of at least 25-50%46.
However, what remains unclear is the extent to which generative AI methodologies enhance clinical translation and progression. This is a crucial consideration, given the huge costs associated with clinical trials. With a number of AI-generated drugs now entering clinical trials, the next few years will prove to be a litmus test for the efficacy of AI tools in drug discovery (Table 1). Modeling shows that it will be improvements in the probability of clinical success that will deliver a significant step change in the economics of R&D46. This point was echoed by Bender and Cortes-Ciriano who found that the decrease in the number of failures, notably clinical failures, is more critical than simply failing more quickly or more cheaply in terms of cost per successful, approved drug (Figure 3)47.
Figure 3: The impact of speed, quality, and cost on the net profit of a drug discovery project, taken from Bender & Cortes-Ciriano, 202147.
Incidentally, since the somewhat skeptical forecast put forward by Bender and Cortes-Ciriano in 2021, there has not been any noticeable surge in the progression of AI-generated drug candidates to the clinic. On the contrary, several AI-centric companies have faced more setbacks and disappointments than success. Exscientia announced in October 2023 that it would be streamlining its pipeline to focus on well-understood development challenges, winding down a Phase 1/2 study of its cancer drug candidate EXS-21546. Similarly, BenevolentAI was forced to lay off almost 50% of its staff and saw a 79% fall in share price after the Phase 2 failure of its Trk inhibitor used for the treatment of eczema48. While it is still too early to tell what effect AI-driven drug discovery will have on clinical success, it is clear that drug discovery is not always as simple as generating highly potent ligands against a single target. As the CEO of Exscientia put it, “If we want to change the probability of success in the clinic, it’s not just better molecules; we also need better translational models.”49 Not only do we need better translational models, but we also need a better understanding of when certain translational models are not necessary, especially from a regulatory perspective (discussed in depth in a previous Alacrita publication).
Fundamentally then, it is essential to develop our human understanding of complex biology if we are to grow in our abilities to successfully model complex biological systems. Understanding how a drug interacts with a target is not simply a question of chemistry, which is well understood in terms of its underlying principles, but also of protein conformational changes, cellular signaling, gene expression, protein modifications, and various other aspects of systems biology that are still poorly understood. Adding the temporal and spatial dimensions to these aspects of biology only further complicates the picture. A consequence of this complexity is that it is much harder to define a finite set of parameters that explain how a drug acts in a cell or organism, making it incredibly challenging to provide meaningful quantitative variables and labels for successful AI implementation.
The attempts that have been made to model the biological response of a drug have thus far relied on the modeling of proxy endpoints from assay data to predict an in vivo, clinical response. For example, the data obtained from assays such as high-throughput screens typically provide a quantitative score of on-target activity as a proxy for efficacy. While HTS proxy data can be somewhat predictive of in vivo efficacy endpoints, data obtained from animal models are more often than not poorly predictive of the human in vivo situation, both in terms of efficacy and safety47. Factoring in the generally poor reproducibility and variability of biological data (compared to chemical data), AI algorithms subjected to biological datasets can therefore suffer from a “garbage in, garbage out” situation, where the problem lies not with the computational method itself but with the data it is trained on. In other words, even if a computational model is well trained on the data provided, and does manage to find an optimal candidate for the proxy endpoints provided, it may not yield a candidate with desired in vivo efficacy and safety. This reaches even further where some clinical endpoints are not adequately predictive of disease.
Pharmaceutical research laboratories are faced with the trade-off of translation risk and execution risk, and in many instances, what can be done is prioritized over what needs to be done: instead of addressing the underlying cause of the disease, existing technologies are used to address a target that remains poorly understood in a human disease context50. That is why in recent years the focus has started to shift towards arguably more clinically relevant (although still inadequate) model systems, such as patient-derived xenograft models, disease-relevant human cell assay systems and artificial tissue or organoid test beds. If generative AI tools in drug discovery are to yield more drugs with improved clinical progression, it will be essential to incorporate human disease-relevant data into the pipeline. Only once the human biology of the target in disease is more clearly understood will the efforts aimed at developing a highly potent and selective pharmaceutical agent using AI lead to faster progression to the clinic, and hopefully, to the market.
4. Future perspectives:
Over the past few decades, therapeutic hypotheses have not developed much beyond the idea that a cure to a disease is just a question of identifying a specific protein in a diseased system and finding a modulator of that protein based on studies in laboratory models. This reductionist approach has indeed resulted in the discovery of hundreds of curative drugs across a range of indications with well-understood pathophysiology, but is not necessarily fruitful when tackling more complex diseases. Whilst games such as GO and chess have been impressively cracked by AI, drug discovery in complex disease has not yet seen the total revolution anticipated from the incorporation of generative AI into the pipeline (although this may just be a question of time). Drug discovery is much more complex than GO, which is a game dictated by a finite set of states and rules that are well defined; the former relies on the careful and contextualized interpretation of high-quality chemical and biological data47. For AI to make significant inroads across the discovery pipeline, it is crucial therefore to break from reductionist approaches that oversimplify human biology, however difficult that may be.
In the coming years, AI implementations in drug discovery will no doubt benefit from the growing wealth of patient-derived data as well as more human-focused preclinical data. With more breadth and depth of data for specific therapeutic areas, use cases, and population sets, AI algorithms will have a stronger grasp of the underlying disease biology and be more predictive of patient outcomes. This of course relies on open access to these datasets, that can often be fragmented in the public domain or privately held. The standardisation and quality of the data will also need to be addressed, given the inconsistency in formatting and the levels of granularity across geographies and industries.
Across the discovery pipeline, AI has already demonstrated its ability to dramatically improve productivity and broaden molecular diversity. What remains to be seen is whether AI-derived drugs can deliver on arguably the most important assessment criteria: improved standard of care and a higher probability of clinical success. Investors clearly have high expectations to see this in the coming years. The field is growing rapidly, with publications and patents related to AI-enabled drug discovery growing by 34% and 17% respectively year-on-year over the last five years46. However, more than 60% of all disclosed targets of AI companies in 2022 were kinases and other well-characterized classes such as GPCRs51. Therefore, as the technology develops, there will be more expectations to see AI tackle more complex diseases with poorly understood biological mechanisms such as neurodegenerative disorders and autoimmune conditions. Until then, the field waits for its first approved AI-derived drug.
References:
- “https://www.morganstanley.com/ideas/ai-drug-discovery.”
- Ayers et al., “Adopting AI in Drug Discovery,” Boston Consulting Group, 2022.
- “The 2023 CPHI Annual Report,” CPHI, 2023.
- Steedman et al., “Unlocking R&D productivity: Measuring the return from pharmaceutical innovation 2018,” Deloitte LLP, 2018.
- Terry & N. Lesser, “Seize the digital momentum: Measuring the return from pharmaceutical innovation 2022,” Deloitte LLP, 2023.
- Bartusiak, “Designing drugs with computers,” Discover, 1981.
- Sadybekov & V. Katritch, “Computational approaches streamlining drug discovery,” Nature, doi: 10.1038/s41586-023-05905-z.
- Kubinyi, “QSAR: Hansch analysis and related approaches,” Weinheim: VcH, 1993
- “https://cloud.google.com/learn/artificial-intelligence-vs-machine-learning#section-1”
- Junshui et al., “Deep Neural Nets as a Method for Quantitative Structure–Activity Relationships” J. of Chem. Inf. Model, 2015, doi: 10.1021/ci500747n.
- Brown et al., “Artificial intelligence in chemistry and drug design,” J Comput Aided Mol Des 2020, doi:10.1007/s10822-020-00317-x.
- “https://openai.com/research/ai-and-compute”
- “https://www.fda.gov/science-research/science-and-research-special-topics/artificial-intelligence-and-machine-learning-aiml-drug-development”.
- Pun et al., “AI-powered therapeutic target discovery,” Trends Pharm. Sci., 2023, doi: 10.1016/j.tips.2023.06.010.
- Pun et al., “Identification of Therapeutic Targets for Amyotrophic Lateral Sclerosis Using PandaOmics – An AI-Enabled Biological Target Discovery Platform,” Front. Aging Neurosci., 2022, doi: 10.3389/fnagi.2022.914017.
- Eccleston, “Phenotypic screen with deep learning finds cardioprotective molecules,” Nat. Rev. Drug Discov., 2022, doi: 10.1038/d41573-022-00132-z.
- Yang et al. “Phenotypic screening with deep learning identifies HDAC6 inhibitors as cardioprotective in a BAG3 mouse model of dilated cardiomyopathy,” Sci. Transl. Med. 2022, doi: 10.1126/scitranslmed.abl5654.
- Batool et al., “A Structure-Based Drug Discovery Paradigm,” Int. J. Mol. Sci., 2019, doi: 10.3390/ijms20112783.
- Zheng et al., “X-ray crystallography over the past decade for novel drug discovery – where are we heading next?,” Expert Opin. Drug Discov., 2016, doi: 10.1517/17460441.2015.1061991.
- Chari & H. Stark, “Prospects and Limitations of High-Resolution Single-Particle Cryo-Electron Microscopy,” Ann. Rev. Biophys., 2023, doi: 10.1146/annurev-biophys-111622-091300.
- “https://www.rcsb.org/stats/growth/growth-released-structures.”
- Jumper et al., “Highly accurate protein structure prediction with AlphaFold,” Nature, 2021, doi: 10.1038/s41586-021-03819-2.
- Callaway, “DeepMind’s AI predicts structures for a vast trove of proteins,” Nature, 2021, doi: 10.1038/d41586-021-02025-4.
- Callaway, “‘The entire protein universe’: AI predicts shape of nearly every known protein,” Nature, 2022, doi: 10.1038/d41586-022-02083-2.
- Karelina et al., “How accurately can one predict drug binding modes using AlphaFold models?,” eLife, 2023, doi: 10.7554/eLife.89386.1.
- “https://ir.recursion.com/news-releases/news-release-details/recursion-bridges-protein-and-chemical-space-massive-protein.”
- Kimani et al., “Discovery of a Novel DCAF1 Ligand Using a Drug–Target Interaction Prediction Model: Generalizing Machine Learning to New Drug Targets,” J. Chem. Inf. Model., 2023, doi: 10.1021/acs.jcim.3c00082.
- Yang et al., “Efficient Exploration of Chemical Space with Docking and Deep Learning,” J. Chem. Theory Comput., 2021, doi: 10.1021/acs.jctc.1c00810.
- “https://news.mit.edu/2023/speeding-drug-discovery-with-diffusion-generative-models-diffdock-0331.”
- Corso et al., “DiffDock: Diffusion Steps, Twists, and Turns for Molecular Docking,” arXiv, 2023, doi: 10.48550/arXiv.2210.01776.
- Gentile et al., “Artificial intelligence–enabled virtual screening of ultra-large chemical libraries with deep docking,” Nat. Protoc., 2022, doi:10.1038/s41596-021-00659-2.
- Clyde et al., “AI-accelerated protein-ligand docking for SARS-CoV-2 is 100-fold faster with no significant change in detection,” Sci. Rep., 2023, doi: 10.1038/s41598-023-28785-9.
- Wong et al., “Benchmarking AlphaFold-enabled molecular docking predictions for antibiotic discovery,” Mol. Sys. Biol., 2022, doi: 10.15252/msb.202211081.
- Kwon et al., “Comprehensive ensemble in QSAR prediction for drug discovery,” BMC bioinf., 2019, doi: 10.1186/s12859-019-3135-4.
- Ma et al., “Deep Neural Nets as a Method for Quantitative Structure–Activity Relationships,” J. Chem. Inf. Model., 2015, doi: 10.1021/ci500747n.
- van Tilborg et al., “Exposing the Limitations of Molecular Machine Learning with Activity Cliffs,” J. Chem. Inf. Model., 2022, doi: 10.1021/acs.jcim.2c01073.
- Cichońska, et al., “Crowdsourced mapping of unexplored target space of kinase inhibitors,” Nat. Commun., 2021, doi: 10.1038/s41467-021-23165-1.
- “https://www.ft.com/content/82071cf2-f0da-432b-b815-606d602871fc.”
- Ivanenkov et al., “Chemistry42: An AI-Driven Platform for Molecular Design and Optimization,” J. Chem. Inf. Model.2023, doi: 10.1021/acs.jcim.2c01191.
- Arnold, “Inside the nascent industry of AI-designed drugs,”Nat. Med., 2023, doi: 10.1038/s41591-023-02361-0.
- Zeng et al., “Repurpose Open Data to Discover Therapeutics for COVID-19 Using Deep Learning,” J. Proteome Res., 2020, doi: 10.1021/acs.jproteome.0c00316.
- Horby et al., “Dexamethasone in Hospitalized Patients with Covid-19,” N. Engl. J. Med., 2020, doi: 10.1056/nejmoa2021436.
- Smith et al., “Expert-Augmented Computational Drug Repurposing Identified Baricitinib as a Treatment for COVID-19,” Front. Pharmacol., 2021, doi: 10.3389/fphar.2021.709856.
- Ahangari et al., “Saracatinib, a Selective Src Kinase Inhibitor, Blocks Fibrotic Responses in Preclinical Models of Pulmonary Fibrosis,” Am. J. Respir. Crit. Care Med., 2022, doi: 10.1164/rccm.202010-3832OC.
- “https://clinicaltrials.gov/study/NCT04598919.”
- “https://wellcome.org/reports/unlocking-potential-ai-drug-discovery.”
- Bender & I. Cortes-Ciriano, “Artificial intelligence in drug discovery: what is realistic, what are illusions? Part 1: Ways to make an impact, and why we are not there yet,” Drug Discovery Today, 2021, doi: 10.1016/j.drudis.2020.12.009.
- “https://www.fiercebiotech.com/biotech/ai-drug-hunter-exscientia-chops-down-rapidly-emerging-pipeline-focus-2-main-oncology.”
- “https://endpts.com/first-ai-designed-drugs-fall-short-in-the-clinic-following-years-of-hype”
- Altshuler, “Vertex Pharmaceuticals: Humanizing drug discovery,” Nature sponsor feature, https://www.nature.com/articles/d42473-020-00303-9.
- Jayatunga, “AI in small-molecule drug discovery: a coming wave?,” Nat. Rev. Drug Discov., 2022, doi: 10.1038/d41573-022-00025-1.
Alacrita Product Development Support
Alacrita is ready to support you through every step of the pharmaceutical product development process, leveraging our scientific, clinical and commercial expertise to help you maximize value at each stage. Please click a service below to learn more or contact us.
Product development services we provide: